Generative AI is at the cutting edge of technological developments that are changing limits and transforming industries in a world where creativity is constantly being pushed to new limits.
It has an amazing capacity for self-content creation; it can produce realistic graphics, music, and even literary works. However, what is generative AI exactly, and how is it different from other types of AI? Let’s set out to explore the latest developments, differences, advantages, and possible drawbacks of this revolutionary technology.
What is Generative ai?
Generative AI, also known as Generative Adversarial Networks, or GANs, is a part of artificial intelligence algorithms that use patterns in existing data to create new material.
It uses neural networks to create unique outputs that mimic human-created material, in contrast to classic AI models that rely on pre-programmed rules.
Also has the potential to transform many industries, including the arts, entertainment, healthcare, and more. It can create realistic visuals, realistic voices, or even whole texts.
The advantages of generative AI:
Generative AI mimics human creativity, enabling artists to go into previously uncharted artistic territory. It is a creative catalyst that inspires creativity and pushes the bounds of imagination in everything from making music to creating visual art.
Generative AI generates synthetic data to help with data enhancement in domains like image processing and natural language understanding. This increases the variety of training datasets and strengthens machine learning models’ resilience and capacity for generalization.
Companies use Generative AI to give customers customized experiences, such as made-to-order product recommendations and specially produced content. This improves customer relationships and encourages greater participation.
Generative AI’s ability to automate content creation tasks streamlines operations and cuts down on the time and resources needed for creative projects. This effectiveness results in quicker production cycles and significant cost savings.
Important differences between other AI approaches and generative AI:
While the two types of learning are common between generative AI and other AI techniques, generative AI is distinguished by its unique features.
Selective vs. Generative Models:
Different models aim to categorize inputs into predetermined categories; in contrast, generative models concentrate on understanding the data’s underlying distribution and producing new samples from it.
Adversarial Training:
The generator and discriminator neural networks of Generative Adversarial Networks (GANs) compete with one another to learn. Through this adversarial training, the generator’s capacity to trick the discriminator is continuously improved, allowing GANs to generate outputs that are incredibly lifelike.
Creative Output production:
Generative AI is an area of specialization that includes text production, visual synthesis, and music composition. Traditional AI models are best at tasks like classification and prediction.
Negative aspects of generative AI:
Generative AI has the potential to be revolutionary, but it also comes with risks and problems.
Ethical Concerns:
The capacity of generative artificial intelligence to produce remarkably lifelike deepfakes gives rise to worries about disinformation, invasions of privacy, and its misuse for evil intent.
Problems with quality control:
Ensuring the authenticity and quality of generated content is still difficult, particularly in crucial sectors like finance and healthcare where biases or mistakes could have serious repercussions.
Resource Intensiveness:
Generative AI model training frequently necessitates enormous volumes of data and computing power, which restricts the models’ scalability and accessibility for smaller businesses or academics with tight budgets.
Most recent fixes and updates:
Researchers and developers are still dedicated to solving the problems that come with generative AI and expanding its potential as it develops. Among the most current fixes and updates are:
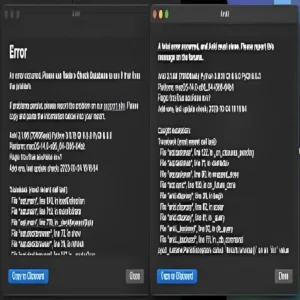
Better Stability and Training Methods:
To improve convergence and sample quality, efforts are being made to develop novel training methods, lessen the effects of mode collapse, and improve the stability of generative AI training.
Error reduction and ethical factors:
Researchers are actively investigating ways to reduce the biases inherent in generative AI models and encourage their ethical application. Creating algorithms that consider fairness and integrating a variety of datasets are two ways to stop preconceptions and prejudices from spreading.
Improving Security and Robustness:
Steps are being taken to strengthen model robustness and protect against adversarial assaults as the use of Generative AI in security-sensitive applications grows. Robust optimization and adversarial training are two strategies that assist strengthen Generative AI systems against future weaknesses.
In summary:
To sum up, generative AI is a major shift in artificial intelligence that opens up fresh possibilities for innovation, creativity, and customisation. Its capacity to create content on its own in a variety of disciplines has enormous potential to transform markets and improve people’s lives.
To fully realize this promise, though, issues with bias, ethics, and security must be resolved while maintaining the highest standards for model robustness and efficiency.
We can steer toward a future where human ingenuity coexists peacefully with intelligent computers and creativity is unbounded by wisely utilizing the revolutionary power of generative AI.